NVIDIA Jetson Orin NX 8 GB
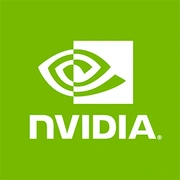
About GPU
The NVIDIA Jetson Orin NX 8GB GPU is a powerful and efficient professional-grade GPU that offers impressive performance for a range of applications. With 8GB of LPDDR5 memory and a memory clockspeed of 1600MHz, the Jetson Orin NX GPU allows for seamless multitasking and quick access to data, making it ideal for data-intensive tasks such as AI inference, robotics, and edge computing.
The 1024 shading units and 256KB L2 cache provide ample processing power for handling complex graphics and computational workloads, while the 20W TDP ensures that the GPU operates efficiently without consuming too much power. This makes it suitable for use in small form factor devices and embedded systems where power consumption is a concern.
The theoretical performance of 1.567 TFLOPS ensures that the Jetson Orin NX GPU can handle demanding computational tasks with ease, making it well-suited for applications such as image and video processing, object detection, and machine learning inference.
Overall, the NVIDIA Jetson Orin NX 8GB GPU is a reliable and versatile solution for professional computing needs. Its combination of high memory capacity, efficient power usage, and impressive performance make it a great choice for developers and engineers working on AI, robotics, and other advanced computing applications. With its robust feature set, this GPU is a valuable asset for anyone looking to take their computing capabilities to the next level.
Basic
Label Name
NVIDIA
Platform
Professional
Launch Date
March 2023
Model Name
Jetson Orin NX 8 GB
Generation
Tegra
Bus Interface
PCIe 4.0 x4
Transistors
Unknown
Tensor Cores
?
Tensor Cores are specialized processing units designed specifically for deep learning, providing higher training and inference performance compared to FP32 training. They enable rapid computations in areas such as computer vision, natural language processing, speech recognition, text-to-speech conversion, and personalized recommendations. The two most notable applications of Tensor Cores are DLSS (Deep Learning Super Sampling) and AI Denoiser for noise reduction.
32
TMUs
?
Texture Mapping Units (TMUs) serve as components of the GPU, which are capable of rotating, scaling, and distorting binary images, and then placing them as textures onto any plane of a given 3D model. This process is called texture mapping.
32
Foundry
Samsung
Process Size
8 nm
Architecture
Ampere
Memory Specifications
Memory Size
8GB
Memory Type
LPDDR5
Memory Bus
?
The memory bus width refers to the number of bits of data that the video memory can transfer within a single clock cycle. The larger the bus width, the greater the amount of data that can be transmitted instantaneously, making it one of the crucial parameters of video memory. The memory bandwidth is calculated as: Memory Bandwidth = Memory Frequency x Memory Bus Width / 8. Therefore, when the memory frequencies are similar, the memory bus width will determine the size of the memory bandwidth.
128bit
Memory Clock
1600MHz
Bandwidth
?
Memory bandwidth refers to the data transfer rate between the graphics chip and the video memory. It is measured in bytes per second, and the formula to calculate it is: memory bandwidth = working frequency × memory bus width / 8 bits.
102.4 GB/s
Theoretical Performance
Pixel Rate
?
Pixel fill rate refers to the number of pixels a graphics processing unit (GPU) can render per second, measured in MPixels/s (million pixels per second) or GPixels/s (billion pixels per second). It is the most commonly used metric to evaluate the pixel processing performance of a graphics card.
12.24 GPixel/s
Texture Rate
?
Texture fill rate refers to the number of texture map elements (texels) that a GPU can map to pixels in a single second.
24.48 GTexel/s
FP16 (half)
?
An important metric for measuring GPU performance is floating-point computing capability. Half-precision floating-point numbers (16-bit) are used for applications like machine learning, where lower precision is acceptable. Single-precision floating-point numbers (32-bit) are used for common multimedia and graphics processing tasks, while double-precision floating-point numbers (64-bit) are required for scientific computing that demands a wide numeric range and high accuracy.
3.133 TFLOPS
FP64 (double)
?
An important metric for measuring GPU performance is floating-point computing capability. Double-precision floating-point numbers (64-bit) are required for scientific computing that demands a wide numeric range and high accuracy, while single-precision floating-point numbers (32-bit) are used for common multimedia and graphics processing tasks. Half-precision floating-point numbers (16-bit) are used for applications like machine learning, where lower precision is acceptable.
783.4 GFLOPS
FP32 (float)
?
An important metric for measuring GPU performance is floating-point computing capability. Single-precision floating-point numbers (32-bit) are used for common multimedia and graphics processing tasks, while double-precision floating-point numbers (64-bit) are required for scientific computing that demands a wide numeric range and high accuracy. Half-precision floating-point numbers (16-bit) are used for applications like machine learning, where lower precision is acceptable.
1.598
TFLOPS
Miscellaneous
SM Count
?
Multiple Streaming Processors (SPs), along with other resources, form a Streaming Multiprocessor (SM), which is also referred to as a GPU's major core. These additional resources include components such as warp schedulers, registers, and shared memory. The SM can be considered the heart of the GPU, similar to a CPU core, with registers and shared memory being scarce resources within the SM.
8
Shading Units
?
The most fundamental processing unit is the Streaming Processor (SP), where specific instructions and tasks are executed. GPUs perform parallel computing, which means multiple SPs work simultaneously to process tasks.
1024
L1 Cache
128 KB (per SM)
L2 Cache
256KB
TDP
20W
Vulkan Version
?
Vulkan is a cross-platform graphics and compute API by Khronos Group, offering high performance and low CPU overhead. It lets developers control the GPU directly, reduces rendering overhead, and supports multi-threading and multi-core processors.
1.3
OpenCL Version
3.0
OpenGL
4.6
DirectX
12 Ultimate (12_2)
CUDA
8.6
Shader Model
6.7
ROPs
?
The Raster Operations Pipeline (ROPs) is primarily responsible for handling lighting and reflection calculations in games, as well as managing effects like anti-aliasing (AA), high resolution, smoke, and fire. The more demanding the anti-aliasing and lighting effects in a game, the higher the performance requirements for the ROPs; otherwise, it may result in a sharp drop in frame rate.
16
Benchmarks
FP32 (float)
Score
1.598
TFLOPS
Compared to Other GPU
FP32 (float)
/ TFLOPS