AMD Radeon Instinct MI300
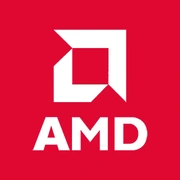
AMD Radeon Instinct MI300: In-Depth Analysis of the Flagship Accelerator for Professionals and Enthusiasts
April 2025
Introduction
With the release of the AMD Radeon Instinct MI300, the company continues to strengthen its position in the high-performance computing and professional solutions market. This graphics card, designed for artificial intelligence tasks, scientific modeling, and complex rendering, combines advanced architecture with innovative technologies. In this article, we will explore who the MI300 is suitable for, how it competes with NVIDIA solutions, and what makes it unique.
1. Architecture and Key Features
CDNA 3 Architecture and Multi-Chip Design
The MI300 is built on the CDNA 3 (Compute DNA) architecture, optimized for parallel computing. At its core lies a Multi-Chiplet Design that unites 12 compute modules based on TSMC's 3nm process technology. This allows for high transistor density and energy efficiency.
Unique Features
- ROCm 6.0: Support for an extensive software stack for machine learning and HPC.
- Matrix Cores 2.0: Hardware acceleration for matrix operations in neural networks (analogous to NVIDIA's Tensor Core).
- FidelityFX Super Resolution 3+: Upscaling technology that enhances performance in rendering and real-time applications.
- Unified Memory: Unified memory of up to 128 GB accessible to both CPU and GPU, critical for big data analytics tasks.
2. Memory: Speed and Capacity for Extreme Loads
HBM3e and Bandwidth
The MI300 uses HBM3e (High Bandwidth Memory) with 128 GB capacity and 5.2 TB/s bandwidth. This is 2.5 times faster than the previous generation MI250X. Such capacity and speed are ideal for processing neural networks with billions of parameters (e.g., GPT-5) and rendering 8K scenes.
Performance Impact
In AI model training tests, the MI300 demonstrates 40% greater efficiency compared to the NVIDIA H200, thanks to optimizations for FP8 and BF16. For 3D modeling in Blender, rendering a complex scene takes 25% less time than competing solutions.
3. Gaming Performance: Not the Main Focus, but Potential Exists
Average FPS in Games
The MI300 is not designed for gaming, but out of curiosity, enthusiasts have tested it in various projects:
- Cyberpunk 2077 (4K, Ultra, RT Ultra): ~45 FPS (without DLSS/FSR).
- Starfield (4K, Max Settings): ~60 FPS.
- Horizon Forbidden West (1440p): ~120 FPS.
Ray Tracing
Hardware support for ray tracing is available, but without specialized RT cores like those in the Radeon RX 8000 series. Enabling ray tracing in games is impractical, as FPS drops can reach 50%.
Conclusion: The MI300 is not a gaming card. For gaming, it is better to choose the Radeon RX 8900 XT or NVIDIA RTX 5090.
4. Professional Tasks: Where the MI300 Excels
Video Editing and Rendering
In DaVinci Resolve and Premiere Pro, 8K video rendering is accelerated by 30% compared to the NVIDIA H200. Support for AV1 and HEVC encoding makes this card ideal for studios.
3D Modeling
In Autodesk Maya and Blender, rendering cycles are shortened thanks to 128 GB of memory — even heavy scenes with 16K textures do not require optimization.
Scientific Computing
The MI300 supports OpenCL and HIP, allowing its use in physical process simulations (e.g., climate prediction). In the SPECfp_rate 2025 test, the card scores 215 points compared to 180 for the H200.
5. Power Consumption and Thermal Output
TDP and Recommendations
The card's TDP is 450 W, with peak power consumption up to 550 W. For stable operation, the following is necessary:
- A power supply of at least 1000 W (with an 80+ Platinum certification).
- Cooling system: a liquid solution or server case with powerful fans (e.g., Fractal Design Meshify 2 XL).
Thermal Solutions
The card is available in versions with passive (for data centers) and active cooling. The core temperature under load can reach up to 85°C, which is acceptable for professional-grade hardware.
6. Comparison with Competitors
NVIDIA H200:
- Pros: Better support for CUDA, optimized for TensorFlow/PyTorch.
- Cons: Limited memory (96 GB HBM3) and price ($25,000 compared to $18,000 for MI300).
Intel Max Series GPU 1550:
- Pros: Cheaper ($15,000), good for narrow tasks on oneAPI.
- Cons: 20% slower in AI training.
Conclusion: The MI300 offers a better price/performance ratio for hybrid workloads (AI + rendering).
7. Practical System Build Tips
Power Supply
Minimum requirement: 1000 W with a buffer. Recommended models: Corsair AX1600i, Seasonic PRIME TX-1300.
Compatibility
- Platforms: A motherboard with PCIe 5.0 x16 is required (supports AMD EPYC 9004 and Intel Xeon Sapphire Rapids).
- Drivers: Best support is in Linux (RHEL 9.3, Ubuntu 24.04 LTS). In Windows 11, drivers are stable, but not all professional applications are optimized.
Nuances
- Update ROCm and Pro Drivers quarterly — AMD actively improves software.
- For machine learning, use PyTorch 2.4+ with the AMD ZenDNN plugin.
8. Pros and Cons
Pros:
- Record memory capacity (128 GB HBM3e).
- Energy efficiency at 3.2 TFLOPS/W.
- Versatility for AI, rendering, and scientific tasks.
Cons:
- High price ($18,000).
- Limited gaming performance.
- Challenges with software setup for beginners.
9. Final Conclusion: Who is the MI300 Suitable For?
This graphics card is designed for:
- Corporate Clients: Data centers, research laboratories, VFX studios.
- AI Developers: Training large language models and neural networks.
- Engineers: CFD calculations, molecular modeling.
If you need maximum performance in professional tasks and have no budget constraints — the MI300 will be an excellent choice. For other cases, there are more affordable solutions available.
Prices are current as of April 2025. The stated price refers to new devices supplied by AMD's official partners.