AMD Instinct MI300X Accelerator
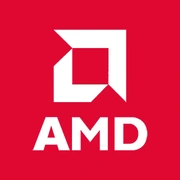
About GPU
The AMD Instinct MI300X Accelerator GPU is a powerhouse of a graphics processing unit designed for desktop use. With a base clock of 1000MHz and a boost clock of 2100MHz, this GPU is built for high-performance computing tasks. The memory size of 192GB, combined with HBM3 memory type and a memory clock of 5200MHz, allows for quick and efficient data processing and storage.
One of the standout features of the AMD Instinct MI300X is its 19456 shading units, which enable complex rendering and calculations to be performed with ease. The 16MB L2 cache further enhances the GPU's ability to handle large amounts of data simultaneously.
With a TDP of 750W, the AMD Instinct MI300X is a high-power GPU that requires a capable cooling system to operate efficiently. However, the theoretical performance of 163.4 TFLOPS makes it a top choice for demanding workloads such as artificial intelligence, scientific research, and high-performance computing.
Overall, the AMD Instinct MI300X Accelerator GPU is a top-of-the-line option for professionals and researchers who require massive computational power for their work. Its impressive specifications and performance capabilities make it an excellent choice for those in need of a reliable and high-performing GPU.
Basic
Label Name
AMD
Platform
Desktop
Launch Date
December 2023
Model Name
Instinct MI300X
Generation
Instinct
Base Clock
1000MHz
Boost Clock
2100MHz
Bus Interface
PCIe 5.0 x16
Memory Specifications
Memory Size
192GB
Memory Type
HBM3
Memory Bus
?
The memory bus width refers to the number of bits of data that the video memory can transfer within a single clock cycle. The larger the bus width, the greater the amount of data that can be transmitted instantaneously, making it one of the crucial parameters of video memory. The memory bandwidth is calculated as: Memory Bandwidth = Memory Frequency x Memory Bus Width / 8. Therefore, when the memory frequencies are similar, the memory bus width will determine the size of the memory bandwidth.
8192bit
Memory Clock
5200MHz
Bandwidth
?
Memory bandwidth refers to the data transfer rate between the graphics chip and the video memory. It is measured in bytes per second, and the formula to calculate it is: memory bandwidth = working frequency × memory bus width / 8 bits.
5300 GB/s
Theoretical Performance
Texture Rate
?
Texture fill rate refers to the number of texture map elements (texels) that a GPU can map to pixels in a single second.
1496 GTexel/s
FP16 (half)
?
An important metric for measuring GPU performance is floating-point computing capability. Half-precision floating-point numbers (16-bit) are used for applications like machine learning, where lower precision is acceptable. Single-precision floating-point numbers (32-bit) are used for common multimedia and graphics processing tasks, while double-precision floating-point numbers (64-bit) are required for scientific computing that demands a wide numeric range and high accuracy.
1300 TFLOPS
FP64 (double)
?
An important metric for measuring GPU performance is floating-point computing capability. Double-precision floating-point numbers (64-bit) are required for scientific computing that demands a wide numeric range and high accuracy, while single-precision floating-point numbers (32-bit) are used for common multimedia and graphics processing tasks. Half-precision floating-point numbers (16-bit) are used for applications like machine learning, where lower precision is acceptable.
81.7 TFLOPS
FP32 (float)
?
An important metric for measuring GPU performance is floating-point computing capability. Single-precision floating-point numbers (32-bit) are used for common multimedia and graphics processing tasks, while double-precision floating-point numbers (64-bit) are required for scientific computing that demands a wide numeric range and high accuracy. Half-precision floating-point numbers (16-bit) are used for applications like machine learning, where lower precision is acceptable.
160.132
TFLOPS
Miscellaneous
Shading Units
?
The most fundamental processing unit is the Streaming Processor (SP), where specific instructions and tasks are executed. GPUs perform parallel computing, which means multiple SPs work simultaneously to process tasks.
19456
L1 Cache
16 KB (per CU)
L2 Cache
16MB
TDP
750W
Benchmarks
FP32 (float)
Score
160.132
TFLOPS
Compared to Other GPU
FP32 (float)
/ TFLOPS